Define "old school programmer".
How much do I love Pascal's Triangle?
The top 100 box office movies of 2008
The final standings of the 2009 baseball season
September temperatures in Oakland: 1969-2009
Tuesday, October 13, 2009
Wednesday, July 29, 2009
Answers to Homework 11
Here are four lists of length n = 16. They correspond to the number of wins, the number of points scored, the number of points allowed. List 4 is List 2 – List 3. (The lists are the number of wins, the number of points scores and the number of points allowed for the teams in the NFC.)
L 1: _12 _12 _11 _10 __9 __9 __9 __9 __9 __8 __8 __7 __6 __4 __2 ____0
L 2: 427 414 391 379 416 427 361 362 375 265 463 339 419 294 232 __268
L 3: 294 329 325 333 289 426 323 265 350 296 393 381 380 392 465 __517
L 4: 133 _85 _66 _46 127 __1 _38 _97 _25 –31 _70 –42 _39 –98 –233 -249
What is the 95% threshold number for rx,y? ___.497___
What is the 99% threshold number for rx,y? ___.623___
Find rx,y for List 1 and List 2: ____.712___ Highest threshold it meets: _99%_
Find rx,y for List 1 and List 3: ____-.802____ Highest threshold it meets:_99%_
Find rx,y for List 1 and List 4: __.907____ Highest threshold it meets:_99%_
Create the ranking list for List 1 and List 4, where 1 is for the highest number and 16 is for the lowest, using the method for ranking ties taught in class.
L 1: _12 _12 11 10 __9 9 _9 _9 _9 ___8 ___8 __7 _6 __4 ___2 ___0
Rank:1.5 1.5 _3 _4 __7 7 _7 _7 _7 10.5 10.5 _12 13 _14 __15 __16
L 4: 133 _85 66 46 127 1 38 97 25 _–31 __70 –42 39 –98 –233 -249
Rank:__1 __4 _6 _7 __2 11 9 _3 10 12 ____5 __13 _8 _14 __15 __16
Rank correlation number for these ranking lists: _.771__
What is the highest threshold it meets? _99%_
L 1: _12 _12 _11 _10 __9 __9 __9 __9 __9 __8 __8 __7 __6 __4 __2 ____0
L 2: 427 414 391 379 416 427 361 362 375 265 463 339 419 294 232 __268
L 3: 294 329 325 333 289 426 323 265 350 296 393 381 380 392 465 __517
L 4: 133 _85 _66 _46 127 __1 _38 _97 _25 –31 _70 –42 _39 –98 –233 -249
What is the 95% threshold number for rx,y? ___.497___
What is the 99% threshold number for rx,y? ___.623___
Find rx,y for List 1 and List 2: ____.712___ Highest threshold it meets: _99%_
Find rx,y for List 1 and List 3: ____-.802____ Highest threshold it meets:_99%_
Find rx,y for List 1 and List 4: __.907____ Highest threshold it meets:_99%_
Create the ranking list for List 1 and List 4, where 1 is for the highest number and 16 is for the lowest, using the method for ranking ties taught in class.
L 1: _12 _12 11 10 __9 9 _9 _9 _9 ___8 ___8 __7 _6 __4 ___2 ___0
Rank:1.5 1.5 _3 _4 __7 7 _7 _7 _7 10.5 10.5 _12 13 _14 __15 __16
L 4: 133 _85 66 46 127 1 38 97 25 _–31 __70 –42 39 –98 –233 -249
Rank:__1 __4 _6 _7 __2 11 9 _3 10 12 ____5 __13 _8 _14 __15 __16
Rank correlation number for these ranking lists: _.771__
What is the highest threshold it meets? _99%_
Tuesday, July 28, 2009
Final homework not accepted late.
The last homework is due tomorrow. I will post the correct answers after class so students can study from them. I will not accept the assignmentafter end of class tomorrow
Monday, July 27, 2009
correlation practice
Did the price of silver correlate well to the price of gold in 2007?
Here are twelve values, each pair taken from a Friday in each month from January to December. The x value is silver and the y value is gold, both in dollars.
Jan 13.45 652.90
Feb 14.49 682.90
Mar 13.03 655.20
Apr 14.01 684.80
May 12.90 654.90
Jun 13.19 654.50
Jul 12.86 664.10
Aug 12.02 673.20
Sep 12.77 715.00
Oct 14.17 783.50
Nov 14.69 808.80
Dec 14.76 838.80
1) What is rx,y? Is it above the 95% confidence level for n = 12? What about the 99% confidence level?
2) If rx,y surpasses either, find the coefficients a and b in the equation yp = ax + b.
3) If rx,y surpasses either, find the month with the lowest absolute residual and the highest absolute residual, which is to say |yp - y| for all twelve months.
Answers in the comments.
Here are twelve values, each pair taken from a Friday in each month from January to December. The x value is silver and the y value is gold, both in dollars.
Jan 13.45 652.90
Feb 14.49 682.90
Mar 13.03 655.20
Apr 14.01 684.80
May 12.90 654.90
Jun 13.19 654.50
Jul 12.86 664.10
Aug 12.02 673.20
Sep 12.77 715.00
Oct 14.17 783.50
Nov 14.69 808.80
Dec 14.76 838.80
1) What is rx,y? Is it above the 95% confidence level for n = 12? What about the 99% confidence level?
2) If rx,y surpasses either, find the coefficients a and b in the equation yp = ax + b.
3) If rx,y surpasses either, find the month with the lowest absolute residual and the highest absolute residual, which is to say |yp - y| for all twelve months.
Answers in the comments.
rank correlation practice
Here is a list of 27 industrialized nations are their ranks, first in infant mortality and second in life expectancy. Being ranked 1st is best and 27th is worst in both situations. Use your calculator to see if the rank correlation for these two rankings has a high enough correlation coefficient for us to be 95% confident of correlation or even 99% confident. Either positive correlation or negative correlation can be used.
countries infant mortality life expectancy
Australia_____ 18 4
Austria_______ 15 16
Belgium_______ 16 19
Canada________ 22 5
Denmark_______ 14 23
Finland_______ 7 21
France________ 6 6
Germany_______ 9 18
Greece________ 24 15
Hong Kong_____ 4 3
Iceland_______ 5 10
Ireland_______ 23 24
Israel________ 12 9
Italy_________ 26 12
Japan_________ 3 1
Netherlands___ 17 17
New Zealand___ 21 11
Norway________ 8 14
Portugal______ 19 25
Singapore_____ 1 2
South Korea___ 13 22
Spain_________ 11 13
Sweden________ 2 7
Switzerland___ 10 8
Taiwan________ 25 27
United Kingdom 20 20
United States_ 27 26
Answers in the comments.
countries infant mortality life expectancy
Australia_____ 18 4
Austria_______ 15 16
Belgium_______ 16 19
Canada________ 22 5
Denmark_______ 14 23
Finland_______ 7 21
France________ 6 6
Germany_______ 9 18
Greece________ 24 15
Hong Kong_____ 4 3
Iceland_______ 5 10
Ireland_______ 23 24
Israel________ 12 9
Italy_________ 26 12
Japan_________ 3 1
Netherlands___ 17 17
New Zealand___ 21 11
Norway________ 8 14
Portugal______ 19 25
Singapore_____ 1 2
South Korea___ 13 22
Spain_________ 11 13
Sweden________ 2 7
Switzerland___ 10 8
Taiwan________ 25 27
United Kingdom 20 20
United States_ 27 26
Answers in the comments.
Friday, July 24, 2009
Practice problems for confidence interval for sigma_x, the standard deviation for the population

We have learned the methods for finding confidence intervals for proportions and averages of populations given similar statistics from samples. There is also a method for estimating the standard deviation of a population and giving a confidence level to that interval.
Let's say we took a sample of 28 scores and got a standard deviation of sx = 16.689, rounded to three places after the decimal. The degrees of freedom is n-1, which in this case is 27. Let's look at the Chi square table at the line that corresponds to d.f. = 27.
____0.995__0.99___0.975__0.95___0.90___||_0.10___0.05___0.025__0.01___0.005
27__11.808 12.879 14.573 16.151 18.114 || 36.741 40.113 43.194 46.963 49.645
The denominators in the formulas shown above are taken from the following columns.
90% confidence: Chi square Big comes from the 0.05 column, Chi square Small comes from the 0.95 column.
95% confidence: Chi square Big comes from the 0.025 column, Chi square Small comes from the 0.975 column.
99% confidence: Chi square Big comes from the 0.005 column, Chi square Small comes from the 0.995 column.
In this example, the formulas would look as follows.
90% confidence interval: sqrt(16.689^2*27/40.113) < sigmax < sqrt(16.689^2*27/16.151)
95% confidence interval: sqrt(16.689^2*27/43.194) < sigmax < sqrt(16.689^2*27/14.573)
99% confidence interval: sqrt(16.689^2*27/49.645) < sigmax < sqrt(16.689^2*27/11.808)
If n-1 is not one of the values in the degrees of freedom chart, use the next lowest number on the list.
Exercise #1: Find the values from the equations listed above, rounded to the nearest thousandth.
Exercise #2: Find the confidence intervals for 90%, 95% and 99% if n = 102 and sigmax = 0.62. Round the answers to two places after the decimal.
Answers in the comments.
Thursday, July 23, 2009
Practice for matched pairs.

Was the price of silver in 2007 significantly different than it was in 2008?
Side by side, we have two lists of prices of silver, the highest price in a given month in 2007, followed by the highest price in that same month in 2008. Take the differences in the prices and find the average and standard deviation. The size of the list is 12, so the degrees of freedom are 11. If we assume we did not know which year showed higher prices when we started this experiment, it make sense to make this a two-tailed test. Just for a change of pace, let us use the 90% confidence level.
Mo.___2007___2008
Jan.__13.45__16.23
Feb.__14.49__19.81
Mar.__13.34__20.67
Apr.__14.01__17.74
May___12.90__18.19
Jun.__13.19__17.50
Jul.__12.86__18.84
Aug.__12.02__15.27
Sep.__12.77__12.62
Oct.__14.17__11.16
Nov.__14.69__10.26
Dec.__14.76__10.66
Find the test statistic t, the threshold from Table A-3 and determine if we should reject H0, which in matched pairs tests is always that mu1 = mu2.
Answers in the comments.
Monday, July 20, 2009
Test results and errors
We do the hypothesis testing because we cannot truly know what reality is, only the test result. If we reject the null hypothesis Ho, we did so because of strong evidence. If there is an error, it is a Type I error. If we set the error threshold at 90% confidence, we expect such errors about 10% of the time. If it is set at 95% confidence, then Type I errors should happen about 5% of the time and at 99% confidence, Type I errors should only happen about 1% of the time.
If we fail to reject H0, the only type of error we can make is called Type II error. The probability of such errors is trickier to compute and we will not work on this problem during this class.
If we fail to reject H0, the only type of error we can make is called Type II error. The probability of such errors is trickier to compute and we will not work on this problem during this class.
Wednesday, July 15, 2009
binomcdp and continuity correction problems
Note: the functions binompdf and binomcdf from the TI-83 and TI-84 are available under slightly different names if you have the Excel spreadsheet program.
TI-83 or TI-84: binompdf(n, p, r) is the same as BINOMDIST(r, n, p, 0) in Excel.
TI-83 or TI-84: binomcdf(n, p, r) is the same as BINOMDIST(r, n, p, 1) in Excel.

Problems:
a) What is the probability of 20 or less successes in 30 independent trials when the probability of success on any one trial is .6?
b) What is the probability of 20 or less successes in 30 independent trials when the probability of success on any one trial is .65?
c) What is the probability of 20 or less successes in 30 independent trials when the probability of success on any one trial is .7?

d) What is the probability of 30 or more successes in 40 independent trials when the probability of success on any one trial is .8?
e) What is the probability of 30 or more successes in 40 independent trials when the probability of success on any one trial is .75?
f) What is the probability of 30 or more successes in 40 independent trials when the probability of success on any one trial is .7?
g) Optional for those with TI-83 calculators or Excel. Find np and nq for each problem and how close the approximations are.
Answers in the comments.
TI-83 or TI-84: binompdf(n, p, r) is the same as BINOMDIST(r, n, p, 0) in Excel.
TI-83 or TI-84: binomcdf(n, p, r) is the same as BINOMDIST(r, n, p, 1) in Excel.

Problems:
a) What is the probability of 20 or less successes in 30 independent trials when the probability of success on any one trial is .6?
b) What is the probability of 20 or less successes in 30 independent trials when the probability of success on any one trial is .65?
c) What is the probability of 20 or less successes in 30 independent trials when the probability of success on any one trial is .7?

d) What is the probability of 30 or more successes in 40 independent trials when the probability of success on any one trial is .8?
e) What is the probability of 30 or more successes in 40 independent trials when the probability of success on any one trial is .75?
f) What is the probability of 30 or more successes in 40 independent trials when the probability of success on any one trial is .7?
g) Optional for those with TI-83 calculators or Excel. Find np and nq for each problem and how close the approximations are.
Answers in the comments.
Wednesday, July 8, 2009
practice problems for binomial distribution
There are problems list at the end of the post through this link, with answers in the comments.
Tuesday, July 7, 2009
Notes on Bayesian probability
You can find notes on Bayesian probability in three posts from last term you can find through this link. Here are a few more practice problems, with answers in the comments.
A) A trait shows up in 20% of the population and the test has a 2% error rate. Find p(error given test positive) and p(error, given test negative).
B) A trait shows up in 10% of the population and the test has a 1% error rate. Find p(error given test positive) and p(error, given test negative).
A) A trait shows up in 20% of the population and the test has a 2% error rate. Find p(error given test positive) and p(error, given test negative).
B) A trait shows up in 10% of the population and the test has a 1% error rate. Find p(error given test positive) and p(error, given test negative).
Saturday, July 4, 2009
Practice problems for homework due 7/6
Take the information of this incomplete contingency table with categories left and right in the columns and yes and no in the rows and fill in the rest of the table using the degrees of freedom.
____________left____right_____row totals
Yes___________25________________75
No____________________50_______
col. totals___90_____________________grand total
Use the information from the completed table to find the following probabilities, both as fractions and as percents rounded to the nearest tenth of a percent.
p-hat(Yes) =
p-hat(Left) =
p-hat(Left and Yes) =
p-hat(Left or Yes) =
p-hat(Left, given Yes) =
p-hat(Yes, given Left) =
State the following complementary sets without using the word NOT, using the categories from above.
NOT (Left) =
NOT (Left or Yes) =
NOT(Right and Yes) =
Answers in the comments.
____________left____right_____row totals
Yes___________25________________75
No____________________50_______
col. totals___90_____________________grand total
Use the information from the completed table to find the following probabilities, both as fractions and as percents rounded to the nearest tenth of a percent.
p-hat(Yes) =
p-hat(Left) =
p-hat(Left and Yes) =
p-hat(Left or Yes) =
p-hat(Left, given Yes) =
p-hat(Yes, given Left) =
State the following complementary sets without using the word NOT, using the categories from above.
NOT (Left) =
NOT (Left or Yes) =
NOT(Right and Yes) =
Answers in the comments.
Tuesday, June 30, 2009
Practice problems for 6/30
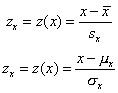
The idea behind z-scores is that we can compare data from completely different numerical data sets by changing the scale to be the distance away from the average, with the new yardstick being the standard deviation. We can take z-scores and the first two pages of the notes to see how common a particular z-score is.
Example: let mux = 63.6 and sigmax = 2.5, which are the average and standard deviation for heights in inches of females.
1) What is the z-score for 67 inches? What percentage of the female population is greater than 67 inches tall?
2) What is the z-score for 64 inches? What percentage of the female population is less than 64 inches tall?

Going the other direction, we have the third page of the notes, which gives z-scores to three decimals that correspond to percentiles in the population. To find the raw score that corresponds to that percentile, use the formulas shown at the left.
3) To the nearest tenth of an inch, what is the height that corresponds to the 96th percentile in U.S. women's heights?
4) To the nearest tenth of an inch, what is the height that corresponds to the 24th percentile in U.S. women's heights?
Answer in the comments.
Labels:
mean,
percentages,
practice problems,
standard deviation,
z-scores
Monday, June 29, 2009
Representing the age at death demographics

The graphical representation of three different groups and their relative frequencies in multiple demographic categories makes the most sense in a bar chart with multiple colors. Red represents the whole population, green represents the Caucasian sub-population and blue represents the African American sub-population.
Click on the picture to see a larger version.
Preview of class for 6/29
Today, we will be discussing distributions of numerical variables. Some variables are evenly distributed through all the values, other skew to have more high values than low, some are the other way around with more low than high.

A lot of data sets have what is called normal distribution, where the most common values are the ones closest to the average, with values much higher or much lower than average being much rarer, and the farther from average those values are, the rarer they become. This curve, known as the bell-shaped curve or the normal curve or the Gaussian curve represents the normal distribution, where the high point shows the density of the values that are near average, and the lower levels at both ends represent the scarcity of values farther away from average.

One of the reasons to find the standard deviation of a set of numbers is to calculate the z-score of a raw value x. This tells us how many standard deviations a value is away from the average. Negative z-scores are for numbers below average and positive z-scores are for values above average. z(x) = 0 only when x = x-bar, the value is exactly at average. The first four pages in the class notes let us change z-scores into proportions, and using these numbers we can talk about the probability of finding values greater than some value x, or the probability of finding values between two values, call them x1 and x2

A lot of data sets have what is called normal distribution, where the most common values are the ones closest to the average, with values much higher or much lower than average being much rarer, and the farther from average those values are, the rarer they become. This curve, known as the bell-shaped curve or the normal curve or the Gaussian curve represents the normal distribution, where the high point shows the density of the values that are near average, and the lower levels at both ends represent the scarcity of values farther away from average.

One of the reasons to find the standard deviation of a set of numbers is to calculate the z-score of a raw value x. This tells us how many standard deviations a value is away from the average. Negative z-scores are for numbers below average and positive z-scores are for values above average. z(x) = 0 only when x = x-bar, the value is exactly at average. The first four pages in the class notes let us change z-scores into proportions, and using these numbers we can talk about the probability of finding values greater than some value x, or the probability of finding values between two values, call them x1 and x2
Sunday, June 28, 2009
Practice problem for standard deviation
Here are two data sets, the number of wins for the teams in the American League as of end of play on Saturday, June 27, and the same statistic in the National League.
Set 1: 46, 42, 41, 41, 34, 41, 38, 36, 31, 31, 40, 40, 38, 31
When you input the data, the size of the data list is 14 and the average is 37 6/7 or 37.857...
===
Set 2: 38, 37, 38, 34, 21, 40, 41, 36, 35, 35, 35, 48, 39, 39, 32, 30
When you input this data set, the size of the set is 16 and the average is 36 1/8 or 36.125 exactly.
Round all answers to one place after the decimal.
a) What is the standard deviation for each set taken as a population, known as sigmax?
b) What is the standard deviation for each set taken as a sample, known as sx?
c) What is the significance of one set having a larger standard deviation than the other set, regardless of whether the measurement is done as a sample or a population?
Answers in the comments.
Set 1: 46, 42, 41, 41, 34, 41, 38, 36, 31, 31, 40, 40, 38, 31
When you input the data, the size of the data list is 14 and the average is 37 6/7 or 37.857...
===
Set 2: 38, 37, 38, 34, 21, 40, 41, 36, 35, 35, 35, 48, 39, 39, 32, 30
When you input this data set, the size of the set is 16 and the average is 36 1/8 or 36.125 exactly.
Round all answers to one place after the decimal.
a) What is the standard deviation for each set taken as a population, known as sigmax?
b) What is the standard deviation for each set taken as a sample, known as sx?
c) What is the significance of one set having a larger standard deviation than the other set, regardless of whether the measurement is done as a sample or a population?
Answers in the comments.
Friday, June 26, 2009
list to frequency table
Here is the list for heights in inches for all students who answered the question in our class survey, put in order from lowest to highest.
60, 60, 60, 61, 62, 62, 63, 63, 64, 65, 65, 66, 66, 66, 66, 66, 66, 66, 66, 68, 68, 69, 69, 69, 70, 70, 71, 71, 71, 72, 72, 74, 76, 77, 78
Only 35 subjects responded, so n = 35.
The frequency table reads as follows
_x____f(x)
60_____3
61_____1
62_____2
63_____2
64_____1
65_____2
66_____8
68_____2
69_____3
70_____2
71_____3
72_____2
74_____1
76_____1
77_____1
78_____1
You can check to see if you have missed any entries by finding the sum of the frequencies, which should be equal to n, which in this case is 35.
60, 60, 60, 61, 62, 62, 63, 63, 64, 65, 65, 66, 66, 66, 66, 66, 66, 66, 66, 68, 68, 69, 69, 69, 70, 70, 71, 71, 71, 72, 72, 74, 76, 77, 78
Only 35 subjects responded, so n = 35.
The frequency table reads as follows
_x____f(x)
60_____3
61_____1
62_____2
63_____2
64_____1
65_____2
66_____8
68_____2
69_____3
70_____2
71_____3
72_____2
74_____1
76_____1
77_____1
78_____1
You can check to see if you have missed any entries by finding the sum of the frequencies, which should be equal to n, which in this case is 35.
frequency table to dot plot and to stem and leaf plot
Here is our frequency table for heights in inches.
_x____f(x)
60_____3
61_____1
62_____2
63_____2
64_____1
65_____2
66_____8
68_____2
69_____3
70_____2
71_____3
72_____2
74_____1
76_____1
77_____1
78_____1
Here is a dot plot using this information.
* * * * * * *
* * * * * * *
* * * * * * *
* * * * * * *
* * * * * * *
* * * * * * * * * * * *
* * * * * * * * * * * * *
* * * * * * * * * * * * * * * * * * *
_____________________________________
6 6 6 6 6 6 6 6 6 6 7 7 7 7 7 7 7 7 7
0 1 2 3 4 5 6 7 8 9 0 1 2 3 4 5 6 7 8
Here is a stem and leaf, with just two stems, 6 and 7.
6 | 000122334556666666688999
7 | 00111224678
We can make a stem and leaf where each of the stems is split into high and low, 60 to 64, 65 to 69, 70 to 74 and 75 to 79.
6 | 000122334
6 | 556666666688999
7 | 00111224
7 | 678
The idea is that the stem and leaf gives the shape of the data. It's okay to have the stem values go from low to high or high to low, as long as the values are consistent.
7 | 00111224678
6 | 000122334556666666688999
Or if we split the decades, we get this.
7 | 678
7 | 00111224
6 | 556666666688999
6 | 000122334
_x____f(x)
60_____3
61_____1
62_____2
63_____2
64_____1
65_____2
66_____8
68_____2
69_____3
70_____2
71_____3
72_____2
74_____1
76_____1
77_____1
78_____1
Here is a dot plot using this information.
* * * * * * *
* * * * * * *
* * * * * * *
* * * * * * *
* * * * * * *
* * * * * * * * * * * *
* * * * * * * * * * * * *
* * * * * * * * * * * * * * * * * * *
_____________________________________
6 6 6 6 6 6 6 6 6 6 7 7 7 7 7 7 7 7 7
0 1 2 3 4 5 6 7 8 9 0 1 2 3 4 5 6 7 8
Here is a stem and leaf, with just two stems, 6 and 7.
6 | 000122334556666666688999
7 | 00111224678
We can make a stem and leaf where each of the stems is split into high and low, 60 to 64, 65 to 69, 70 to 74 and 75 to 79.
6 | 000122334
6 | 556666666688999
7 | 00111224
7 | 678
The idea is that the stem and leaf gives the shape of the data. It's okay to have the stem values go from low to high or high to low, as long as the values are consistent.
7 | 00111224678
6 | 000122334556666666688999
Or if we split the decades, we get this.
7 | 678
7 | 00111224
6 | 556666666688999
6 | 000122334
Labels:
dot plots,
frequency tables,
stem and leaf plots
Five number summary and box and whiskers plot
The five number summary does not list all the information, but instead lists the low value, Q1, Q2, Q3 and the high value. The Q's stand for quartile, which means Q1 is the split for the low 25% of the data, Q2 is the median and Q3 is the split for the high half of the data. You can think of Q1 and Q2 as the medians of the low half and the high half, respectively. The interquartile range or IQR is the distance from the third quartile to the first, which is Q3 - Q1.
Let's look at the list.
7 | 678
7 | 00111224
6 | 556666666688999
6 | 000122334
With 35 entries, the middle entry is the 18th, either counting from top to bottom or bottom to top. That is the 6 marked in red and bold, so Q2 = 66.
There are 17 entries in the top half and 17 in the low half, so the position of the high and low quartiles is 9 away from the top and 9 away from the bottom, respectively. These are marked in bold and blue, with Q1 = 64 and Q3 = 71.
The five number summary is as follows
High = 78
Q3 = 71
Q2 = 66
Q1 = 64
Low = 60
Now we draw the box and whiskers in three steps, shown in the picture.

The first step is to draw the box between the values for the first and third quartiles, with a dotted line at the second quartile. We also can put dots to mark the high and low values.
The next step is computing the Interquartile Range, IQR = Q3 - Q1, which in this case is 71-64 = 7. We mark boundaries called the threshold which will be 1.5 times IQR above the third quartile and 1.5 time IQR below the first quartile. In our case, this would be at 71+ 1.5*7 = 81.5 and 64 - 1.5*7 = 63.5.
The third step is checking for outliers, If the high and low values are within these thresholds, the whiskers extend to the high value to the right and the low value to the left. (Box and whiskers can also be drawn vertically, so change the directions to high an dlow in that case.) If all the data is inside, the whiskers extend all the way to high and low. If there is data outside, those count as outliers and the whiskers are drawn to the highest data inside the high threshold and the lowest data inside the low threshold. One this has been done, we erase the thresholds.
Let's look at the list.
7 | 678
7 | 00111224
6 | 556666666688999
6 | 000122334
With 35 entries, the middle entry is the 18th, either counting from top to bottom or bottom to top. That is the 6 marked in red and bold, so Q2 = 66.
There are 17 entries in the top half and 17 in the low half, so the position of the high and low quartiles is 9 away from the top and 9 away from the bottom, respectively. These are marked in bold and blue, with Q1 = 64 and Q3 = 71.
The five number summary is as follows
High = 78
Q3 = 71
Q2 = 66
Q1 = 64
Low = 60
Now we draw the box and whiskers in three steps, shown in the picture.
The first step is to draw the box between the values for the first and third quartiles, with a dotted line at the second quartile. We also can put dots to mark the high and low values.
The next step is computing the Interquartile Range, IQR = Q3 - Q1, which in this case is 71-64 = 7. We mark boundaries called the threshold which will be 1.5 times IQR above the third quartile and 1.5 time IQR below the first quartile. In our case, this would be at 71+ 1.5*7 = 81.5 and 64 - 1.5*7 = 63.5.
The third step is checking for outliers, If the high and low values are within these thresholds, the whiskers extend to the high value to the right and the low value to the left. (Box and whiskers can also be drawn vertically, so change the directions to high an dlow in that case.) If all the data is inside, the whiskers extend all the way to high and low. If there is data outside, those count as outliers and the whiskers are drawn to the highest data inside the high threshold and the lowest data inside the low threshold. One this has been done, we erase the thresholds.
Standard deviation using the TI-30x IIs
With this list, we can use the TI-30x IIs to get the average and both standard deviations, sx, the standard deviation for a sample and sigmax, the standard deviation for a population.
The buttons to press will be written in red.
Here is a data set we will input.
60, 60, 60, 61, 62, 62, 63, 63,64, 65, 65, 66, 66, 66, 66, 66, 66, 66, 66,
68, 68, 69, 69, 69, 70, 70, 71, 71, 71, 72, 72, 74, 76, 77, 78
[2nd][DATA][ENTER] This puts the calculator in one variable mode.
[2nd][DATA][LEFT][ENTER] This clears the data.
[DATA]
X1= 60 [DOWN]
FRQ= 3 [DOWN]X2= 61 [DOWN]
FRQ= 1 [DOWN]X3= 62 [DOWN]
FRQ= 2 [DOWN]X4= 63 [DOWN]
FRQ= 2 [DOWN]X5= 64 [DOWN]
FRQ= 1 [DOWN]X6= 65 [DOWN]
FRQ= 2 [DOWN]X7= 66 [DOWN]
FRQ= 8 [DOWN]X8= 68 [DOWN]
FRQ= 2 [DOWN]X9= 69 [DOWN]
FRQ= 3 [DOWN]X10= 70 [DOWN]
FRQ= 2 [DOWN]X11= 71 [DOWN]
FRQ= 3 [DOWN]X12= 72 [DOWN]
FRQ= 2 [DOWN]X13= 74 [DOWN]
FRQ= 1 [DOWN]X14= 76 [DOWN]
FRQ= 1 [DOWN]X15= 77 [DOWN]
FRQ= 1 [DOWN]X16= 78 [DOWN]
FRQ= 1 [DOWN]
[STATVAR] This shows the statistics variables.
n x-bar sx sigmax
35[RIGHT]n x-bar sx sigmax
67.37142857[RIGHT]n x-bar sx sigmax
4.747046848[RIGHT]n x-bar sx sigmax
4.67870455[RIGHT]
The buttons to press will be written in red.
Here is a data set we will input.
60, 60, 60, 61, 62, 62, 63, 63,64, 65, 65, 66, 66, 66, 66, 66, 66, 66, 66,
68, 68, 69, 69, 69, 70, 70, 71, 71, 71, 72, 72, 74, 76, 77, 78
[2nd][DATA][ENTER] This puts the calculator in one variable mode.
[2nd][DATA][LEFT][ENTER] This clears the data.
[DATA]
X1= 60 [DOWN]
FRQ= 3 [DOWN]X2= 61 [DOWN]
FRQ= 1 [DOWN]X3= 62 [DOWN]
FRQ= 2 [DOWN]X4= 63 [DOWN]
FRQ= 2 [DOWN]X5= 64 [DOWN]
FRQ= 1 [DOWN]X6= 65 [DOWN]
FRQ= 2 [DOWN]X7= 66 [DOWN]
FRQ= 8 [DOWN]X8= 68 [DOWN]
FRQ= 2 [DOWN]X9= 69 [DOWN]
FRQ= 3 [DOWN]X10= 70 [DOWN]
FRQ= 2 [DOWN]X11= 71 [DOWN]
FRQ= 3 [DOWN]X12= 72 [DOWN]
FRQ= 2 [DOWN]X13= 74 [DOWN]
FRQ= 1 [DOWN]X14= 76 [DOWN]
FRQ= 1 [DOWN]X15= 77 [DOWN]
FRQ= 1 [DOWN]X16= 78 [DOWN]
FRQ= 1 [DOWN]
[STATVAR] This shows the statistics variables.
n x-bar sx sigmax
35[RIGHT]n x-bar sx sigmax
67.37142857[RIGHT]n x-bar sx sigmax
4.747046848[RIGHT]n x-bar sx sigmax
4.67870455[RIGHT]
Wednesday, June 24, 2009
Practice problems for frequency tables
x___f(x)
20__5
19__6
18__7
17__6
16__4
15__4
14__3
13__2
12__1
11__2
Using this data set written as a frequency table, find the average x-bar, n, the median and the mode.
Answers in the comments.
20__5
19__6
18__7
17__6
16__4
15__4
14__3
13__2
12__1
11__2
Using this data set written as a frequency table, find the average x-bar, n, the median and the mode.
Answers in the comments.
Notes for 6/24
I'm going to be putting previews of notes for class before the class, then fleshing in the details and possibly adding more or taking away topics from the list, depending on how far we get.
Wednesday's topics:
Frequency tables: categorical and numerical
Five number summaries
Box and whisker plots
stem and leaf plots
Histograms (also known as bar charts)
Pareto charts
Pie charts
Wednesday's topics:
Frequency tables: categorical and numerical
Five number summaries
Box and whisker plots
stem and leaf plots
Histograms (also known as bar charts)
Pareto charts
Pie charts
Notes for 6/23
Types of numerical data
Coded numerical: Usually, with numbers there is a meaning we can give to the ideas of "more" and "less". With coded data, we don't necessarily have that. Examples are zip codes, social security numbers and driver's licenses. Finding the average zip code of a group of people is meaningless, as is the mid-range and median. Finding the mode means that is the most popular of the possible zip codes, and that does give valuable information.
Ordinal data: Here, the idea of a > b has meaning, but the distance between units isn't the same. In a ranking system, it's better to be first than it is to be second, but we can't say how much better, and we don't know if the difference between first and second is the same as the distance between second and third.
Often, when we switch from an ordered categorical system like grades (A, B, C, D, F) to the numbers used for grade points (4.0, 3.0, 2.0, 1.0, 0.0), the choice of what numbers to use is arbitrary. Is the distance from an A to B really the same as the distance from a C to a D? Is getting an A in one class and a C in another really the same as getting two Bs, since both would be a 3.0 Grade Point Average (GPA). How about 2 As and a D, which is 3.0, or 3 As and an F? Should all those situations be counted the same way?
The feeling of this instructor is that it should not. Again, like coded data, ordinal data can use some of the measures of center, like median and mode, but average and mid-range do not give useful information.
Interval data: This is the minimum requirement need for mean to make sense, the idea that the distance between two numbers, a - b, has a consistent meaning, like degrees in temperature readings or the number of strokes taken to complete a round of golf. In these system, the number zero does not mean the complete absence of a thing, so it dividing one number by another from the data set doesn't give meaningful information, but taking and average is about adding values together and dividing by the number of values, so an average temperature or an average of the scores in four rounds of golf does produce a useful statistic.
Rational data: This is data where not only a - b means something, but also a/b. The difference between interval and rational data is the meaning of the number zero. If zero indicates the complete lack of a thing, then we can talk about something between twice as much as another thing, or 10% less. A lot of numerical systems of measurement are rational, but not all.
Measures of center
Mode
Type of data: any data can be used, but only if there are duplicate values on the list.
Method: Find the most common value. If there is a tie for most common, there can be more than one mode.
Median
Type of data: numerical or ordered categorical
Method: Put the values in order and find the "middle value" which is the value in position (n+1)/2. If n is odd, there is a single median value on the list. If n is even, there are two middle values on the list, and if numerical, take the average of the two. If the data is categorical and the two values aren't the same, the median lies between two categories.
Mean (average)
Type of data: numerical
Method: add up all the numbers and divide by n (or N), the number of things on the list.
Mid-range
Type of data: numerical
Method: (high + low)/2
Coded numerical: Usually, with numbers there is a meaning we can give to the ideas of "more" and "less". With coded data, we don't necessarily have that. Examples are zip codes, social security numbers and driver's licenses. Finding the average zip code of a group of people is meaningless, as is the mid-range and median. Finding the mode means that is the most popular of the possible zip codes, and that does give valuable information.
Ordinal data: Here, the idea of a > b has meaning, but the distance between units isn't the same. In a ranking system, it's better to be first than it is to be second, but we can't say how much better, and we don't know if the difference between first and second is the same as the distance between second and third.
Often, when we switch from an ordered categorical system like grades (A, B, C, D, F) to the numbers used for grade points (4.0, 3.0, 2.0, 1.0, 0.0), the choice of what numbers to use is arbitrary. Is the distance from an A to B really the same as the distance from a C to a D? Is getting an A in one class and a C in another really the same as getting two Bs, since both would be a 3.0 Grade Point Average (GPA). How about 2 As and a D, which is 3.0, or 3 As and an F? Should all those situations be counted the same way?
The feeling of this instructor is that it should not. Again, like coded data, ordinal data can use some of the measures of center, like median and mode, but average and mid-range do not give useful information.
Interval data: This is the minimum requirement need for mean to make sense, the idea that the distance between two numbers, a - b, has a consistent meaning, like degrees in temperature readings or the number of strokes taken to complete a round of golf. In these system, the number zero does not mean the complete absence of a thing, so it dividing one number by another from the data set doesn't give meaningful information, but taking and average is about adding values together and dividing by the number of values, so an average temperature or an average of the scores in four rounds of golf does produce a useful statistic.
Rational data: This is data where not only a - b means something, but also a/b. The difference between interval and rational data is the meaning of the number zero. If zero indicates the complete lack of a thing, then we can talk about something between twice as much as another thing, or 10% less. A lot of numerical systems of measurement are rational, but not all.
Measures of center
Mode
Type of data: any data can be used, but only if there are duplicate values on the list.
Method: Find the most common value. If there is a tie for most common, there can be more than one mode.
Median
Type of data: numerical or ordered categorical
Method: Put the values in order and find the "middle value" which is the value in position (n+1)/2. If n is odd, there is a single median value on the list. If n is even, there are two middle values on the list, and if numerical, take the average of the two. If the data is categorical and the two values aren't the same, the median lies between two categories.
Mean (average)
Type of data: numerical
Method: add up all the numbers and divide by n (or N), the number of things on the list.
Mid-range
Type of data: numerical
Method: (high + low)/2
Labels:
mean,
measures of center,
median,
mid range,
mode
Tuesday, June 23, 2009
practice problems for homework 1
1. Find the frequencies and relative frequencies for the ethnicity variable. Round the relative frequencies by the +/- 0.1% rule.
2. Using just the men's heights, find the following statistics from the sample. Round non-integer answers to the nearest tenth.
n =
x-bar =
mode =
median =
maximum value =
minimum value =
mid-range =
Answer in the comments.
2. Using just the men's heights, find the following statistics from the sample. Round non-integer answers to the nearest tenth.
n =
x-bar =
mode =
median =
maximum value =
minimum value =
mid-range =
Answer in the comments.
summer survey data
Here is the data from the summer survey. There has been some sorting, so the subject numbers will be different from the numbers on your list, but the general data is the same.
The columns are
Subject #
Gender
Ethnicity
Height in inches
Age
L/R handedness
Difficulty of class
GPA
Hours of sleep
Major
Math Opinion
_1 F African_ 64 30-39___ L 5 3.10 6___ BF___ B
_2 F AfroAm__ 66 20-29___ R 4 2.00 ____ CESM_ E
_3 F AfroAm__ 60 20-29___ R 2 3.19 6.5_ HE___ C
_4 F AfroAm__ 66 30-39___ R 4 3.00 4.5_ BF___ C
_5 F AfroAm__ 74 30-39___ R 3 2.90 8.5_ AH___ C
_6 F Asian___ 61 19&under R 5 4.00 7___ Und__ B
_7 F Asian___ 62 19&under R 4 3.16 7___ BF___ B
_8 F Asian___ __ 20-29___ R 3 3.59 7.5_ CESM_ C
_9 F Asian___ 66 20-29___ R 3 ____ ____ BF___ C
10 F Asian___ 65 20-29___ R 2 3.70 5___ HE___ B
11 F Asian___ 60 30-39___ R 4 4.00 8___ SS___ D
12 F Asian___ 63 40-49___ R 4 ____ 8___ Und__ B
13 F AsianAm_ 60 19&under L 3 2.66 10__ CESM_ D
14 F AsianAm_ 62 20-29___ L 5 2.89 6___ Other D
15 F AsianAm_ 66 40-49___ R 5 3.85 7___ HE___ E
16 F EuroAm__ 69 20-29___ R 3 4.00 8.5_ HE___ D
17 F Hispanic 66 20-29___ R 4 3.50 7___ SS___ A
18 F Other___ 66 20-29___ R 4 3.50 6.5_ Other B
19 M African_ 68 30-39___ R 3 3.78 5.5_ HE___ E
20 M AfroAm__ 72 19&under R 3 2.45 10.5 CESM_ B
21 M AfroAm__ 71 20-29___ R 3 2.36 7___ Other C
22 M AfroAm__ 78 50&over_ R 4 3.10 7___ AH___ E
23 M Asian___ 72 19&under R 3 2.50 7___ BF___ B
24 M Asian___ 69 19&under R 2 3.52 7___ CESM_ E
25 M Asian___ 70 20-29___ R 4 2.85 7___ Other C
26 M Asian___ __ 20-29___ R 3 3.00 7.5_ BF___ C
27 M Asian___ __ 20-29___ R 3 ____ 7___ BF___ D
28 M AsianAm_ 66 19&under R 3 3.00 6___ Und__ C
29 M EuroAm__ 76 19&under R 3 3.60 7___ Und__ C
30 M EuroAm__ 71 50&over_ L 1 3.76 5___ CESM_ E
31 M European 69 20-29___ R 4 ____ 9___ SS___ B
32 M Hispanic 63 19&under R 2 3.40 8___ AH___ E
33 M Hispanic 77 19&under R 1 ____ 5___ CESM_ E
34 M Hispanic 66 20-29___ R 3 2.67 6.5_ HE___ C
35 M Hispanic 68 30-39___ R 3 3.50 7.5_ Other E
36 M Other___ 70 19&under R 5 3.83 9___ Und__ E
37 M Other___ 65 20-29___ R 5 3.87 4___ Other C
38 M Other___ 71 20-29___ R 5 3.00 6.5_ Und__ C
n= 38 38_____ 35 38_____ 38 38 33_ 36__ 38___ 38
The columns are
Subject #
Gender
Ethnicity
Height in inches
Age
L/R handedness
Difficulty of class
GPA
Hours of sleep
Major
Math Opinion
_1 F African_ 64 30-39___ L 5 3.10 6___ BF___ B
_2 F AfroAm__ 66 20-29___ R 4 2.00 ____ CESM_ E
_3 F AfroAm__ 60 20-29___ R 2 3.19 6.5_ HE___ C
_4 F AfroAm__ 66 30-39___ R 4 3.00 4.5_ BF___ C
_5 F AfroAm__ 74 30-39___ R 3 2.90 8.5_ AH___ C
_6 F Asian___ 61 19&under R 5 4.00 7___ Und__ B
_7 F Asian___ 62 19&under R 4 3.16 7___ BF___ B
_8 F Asian___ __ 20-29___ R 3 3.59 7.5_ CESM_ C
_9 F Asian___ 66 20-29___ R 3 ____ ____ BF___ C
10 F Asian___ 65 20-29___ R 2 3.70 5___ HE___ B
11 F Asian___ 60 30-39___ R 4 4.00 8___ SS___ D
12 F Asian___ 63 40-49___ R 4 ____ 8___ Und__ B
13 F AsianAm_ 60 19&under L 3 2.66 10__ CESM_ D
14 F AsianAm_ 62 20-29___ L 5 2.89 6___ Other D
15 F AsianAm_ 66 40-49___ R 5 3.85 7___ HE___ E
16 F EuroAm__ 69 20-29___ R 3 4.00 8.5_ HE___ D
17 F Hispanic 66 20-29___ R 4 3.50 7___ SS___ A
18 F Other___ 66 20-29___ R 4 3.50 6.5_ Other B
19 M African_ 68 30-39___ R 3 3.78 5.5_ HE___ E
20 M AfroAm__ 72 19&under R 3 2.45 10.5 CESM_ B
21 M AfroAm__ 71 20-29___ R 3 2.36 7___ Other C
22 M AfroAm__ 78 50&over_ R 4 3.10 7___ AH___ E
23 M Asian___ 72 19&under R 3 2.50 7___ BF___ B
24 M Asian___ 69 19&under R 2 3.52 7___ CESM_ E
25 M Asian___ 70 20-29___ R 4 2.85 7___ Other C
26 M Asian___ __ 20-29___ R 3 3.00 7.5_ BF___ C
27 M Asian___ __ 20-29___ R 3 ____ 7___ BF___ D
28 M AsianAm_ 66 19&under R 3 3.00 6___ Und__ C
29 M EuroAm__ 76 19&under R 3 3.60 7___ Und__ C
30 M EuroAm__ 71 50&over_ L 1 3.76 5___ CESM_ E
31 M European 69 20-29___ R 4 ____ 9___ SS___ B
32 M Hispanic 63 19&under R 2 3.40 8___ AH___ E
33 M Hispanic 77 19&under R 1 ____ 5___ CESM_ E
34 M Hispanic 66 20-29___ R 3 2.67 6.5_ HE___ C
35 M Hispanic 68 30-39___ R 3 3.50 7.5_ Other E
36 M Other___ 70 19&under R 5 3.83 9___ Und__ E
37 M Other___ 65 20-29___ R 5 3.87 4___ Other C
38 M Other___ 71 20-29___ R 5 3.00 6.5_ Und__ C
n= 38 38_____ 35 38_____ 38 38 33_ 36__ 38___ 38
Notes for 6/22
Much of statistics deals with data sets. A data set can either be a population, which means it contains everyone in a particular group we are interested in, or it can be a sample, which means a subset of a population. For instance, if everyone in class shows up on the day of a quiz, I could consider that the population of students in the class and the scores on the quiz could be the variable we are collecting. On the other hand, the class could be considered a sample of all students at Laney, or all students at Laney taking statistics, or all students in classes that start at 12:15. The decision on whether it is a sample or a population in many cases can be considered arbitrary, which means that someone made a decision. Arbiter means judge, and some arbitrary decisions are based on simple personal preference while other arbitrary decisions may be based on pre-set rules.

In statistics, there are some symbols that are reserved, which means a particular letter cannot be used to mean just anything. The first two such letters are N and n, which mean the size of a population and the size of a sample, respectively. The first kind of data we have dealt with is categorical data, where the answers to the questions are not numerical. How often a particular answer shows up in the population is called the frequency, denoted by F in a population or f in a sample. It would be nice if capital letters always meant population and lowercase letters always meant sample, but that is not the case. We also have relative frequency, which is p in a population and p-hat in a sample.
Note: the text editor for the blog doesn't allow for fancy marks on letters or even Greek letters, so in some cases when typing, the symbols will have to be replaced with words like p-hat or x-bar, which is the way they are pronounced.
There is also the situation of subscripts and superscripts. On the blog, it is possible to show subscripts, like x3, but if we want to square a number, the text editor doesn't allow making a small number that floats above the line, so instead we will use x^2. The symbol "^" is also used on your calculator to indicate raising a number to a power.
Frequencies are always whole numbers, either positive integers or zero. Relative frequencies are proportions, numbers between 0 and 1, inclusively. We could write these as fractions, but we often use decimals or percents, which leads to rounding.
Rounding proportions
In gneral, people like to use percents when talking about proportions because 23% looks like a whole number, though it really isn't. 23% is the same as 23/100 or 0.23. When dealing with large proportions, which is to say proportions over 1%, percent will be the standard. How far we round the number will depend on the sum of all relative frequencies.
For example, if we have four categories and each category has a relative frequency of 1/4, we could write 25% for each. If we add up all relative frequencies and we don't round, the sum will be exactly 1 or 100%.
25%+25%+25%+25= 100%
If instead we have eight categories and each has a relative frequency of 1/8, rounding to the nearest percent gives us 13%. (Without rounding 1/8 = .125, so we would round up.)
13%+13%+13%+13%+13%+13%+13%+13%=104%
If the sum is more than one tenth of one percent away from 100%, we need to round to more places. In this case 1/8 = 12.5% exactly so if we write the proportions to the nearest tenth of a percent, we get
12.5%+12.5%+12.5%+12.5%+12.5%+12.5%+12.5%+12.5%=100%
With 1/3 = .333......, we don't get so lucky.
33%+33%+33%=99% (Close, but not 100%.)
33.3%+33.3%+33.3%=99.9% (Still not exactly right.)
33.33%+33.33%+33.33%=99.99% (Still not exactly right, and it never will be.)
Since in some cases, rounding will always produce some error, we make the arbitrary decision that being within one tenth of one percent is close enough, which means between 99.9% and 100.1%, inclusive. So with in this case, we should round to the nearest tenth of a percent.
Scales based on powers of 10: The most famous scale base on powers of ten in percentage, which really means "per 100". It is much more common to see "53% of the people agree with the president's plan" than ".53 of the people..." or "53 out of every 100 people...". Technically, all those phrases are saying the same thing, but percentage is the most popular.
One of the places where decimals are used for proportions is in the sports pages. A batting average in baseball (hits/at bats) is given as a percent to three decimal place, and likewise winning proportions (win/total games) are written as .xxx. If a batter has 27 hits in 92 at-bats, the batting average 27/92 = .293478261... is shortened to .293 and pronounced "two ninety three". Likewise, a team who has won 17 games and lost 5 will have a winning proportion of 17/22 = .77272727... = .773, and often stated as "team has a winning percentage of seven seventy three." Technically, this is a mistake, because "percentage" means out or 100. The correct word from the dictionary, which no one ever uses, is "permillage", which means out of 1,000. The team in question would have a winning percentage of 77/100, and a winning permillage of 773/1000.
In both of the cases from the sports pages, the greater number of place after the decimal is used to break ties. For example, a team with 14 wins and 4 losses is at .778, which is better than 17 wins and 5 losses, while 20 wins and 6 losses is at .769, so is slightly worse.
To get a number based on a power of 10 scale, you take the proportion and multiply by the power of ten, so it is either p*scale or p-hat*scale, depending on population or sample. Besides greater precision for breaking ties, sometimes we need greater precision because the proportions are so small.
When I ask a class what is the legal limit for blood alcohol while driving, invariably someone will say "point oh eight" and most people will agree. But .08 is wrong. .08 = 8%, and the correct answer is .08% = .0008. I don't blame the students. The number is badly represented and it is an easy mistake to make. Let's take a look at the number on other scales of 10.
.08 out of 100 is the same as
.8 out of 1,000 0r
8 out of 10,000 or
80 out of 100,000
80 parts out of 100,000 is a tiny proportion. To give an idea, ounce of pure alcohol mixed into ten gallons of blood would give you 78 parts out of 100,000, and most people have between a half gallon and a gallon and a half of blood in their body, between 4 and 12 pints. The amount of alcohol in a person's blood stream that is over the legal limit is about the same amount of alcohol as found in a capful of mouthwash used after brushing your teeth.
We will be dealing with much smaller proportions later in the class, where there are things that can be hazardous to your health at ranges measure in parts per billion, but for now, we will look at the per 100,000 scale for another type of statistic, measurements of mortality rates.
Here are the number of homicides in some local cities in 2007.
Oakland: 124 homicides
Richmond: 28 homicides
San Francisco: 98 homicides
Clearly, comparing these numbers is misleading, because we know these cities have very different numbers of citizens, so the standard way to measure these statistics is the per 100,000 population scale, which we find by the formula
f/n* scale
which in this case is
(# of homicides)/(city population) * 100,000
Oakland's population in 2007 is estimated at 415,000, Richmond at 106,000 and San Francisco at 825,000, so the murder rates on this standard scale are as follows
Oakland: 124/415000 * 100000 = 29.9
Richmond: 28/106000 * 100000 = 26.4
San Francisco: 98/825000 * 100000 = 11.9
So even though more people were murdered in San Francisco than in Richmond in 2007, the murder rate in Richmond was over twice as high, because Richmond has barely 1/8 of the population of San Francisco. (note: The trends for the three cities this decade are going in different directions. Oakland's murder rate is on the rise, while Richmond's is falling and San Francisco's has stayed about the same.)
Calculating proportions (probabilities): There are times when we will need to find new proportions from information previously calculated, either adding and subtracting old numbers or multiplying or dividing. It's best to use the fractional forms of the data when available, then round the answers after using the exact numbers instead of using answers that might have been rounded. Every time you use a rounded answer in a calculation, there is a change to increase the rounding error even more.

In statistics, there are some symbols that are reserved, which means a particular letter cannot be used to mean just anything. The first two such letters are N and n, which mean the size of a population and the size of a sample, respectively. The first kind of data we have dealt with is categorical data, where the answers to the questions are not numerical. How often a particular answer shows up in the population is called the frequency, denoted by F in a population or f in a sample. It would be nice if capital letters always meant population and lowercase letters always meant sample, but that is not the case. We also have relative frequency, which is p in a population and p-hat in a sample.

There is also the situation of subscripts and superscripts. On the blog, it is possible to show subscripts, like x3, but if we want to square a number, the text editor doesn't allow making a small number that floats above the line, so instead we will use x^2. The symbol "^" is also used on your calculator to indicate raising a number to a power.
Frequencies are always whole numbers, either positive integers or zero. Relative frequencies are proportions, numbers between 0 and 1, inclusively. We could write these as fractions, but we often use decimals or percents, which leads to rounding.
Rounding proportions
In gneral, people like to use percents when talking about proportions because 23% looks like a whole number, though it really isn't. 23% is the same as 23/100 or 0.23. When dealing with large proportions, which is to say proportions over 1%, percent will be the standard. How far we round the number will depend on the sum of all relative frequencies.
For example, if we have four categories and each category has a relative frequency of 1/4, we could write 25% for each. If we add up all relative frequencies and we don't round, the sum will be exactly 1 or 100%.
25%+25%+25%+25= 100%
If instead we have eight categories and each has a relative frequency of 1/8, rounding to the nearest percent gives us 13%. (Without rounding 1/8 = .125, so we would round up.)
13%+13%+13%+13%+13%+13%+13%+13%=104%
If the sum is more than one tenth of one percent away from 100%, we need to round to more places. In this case 1/8 = 12.5% exactly so if we write the proportions to the nearest tenth of a percent, we get
12.5%+12.5%+12.5%+12.5%+12.5%+12.5%+12.5%+12.5%=100%
With 1/3 = .333......, we don't get so lucky.
33%+33%+33%=99% (Close, but not 100%.)
33.3%+33.3%+33.3%=99.9% (Still not exactly right.)
33.33%+33.33%+33.33%=99.99% (Still not exactly right, and it never will be.)
Since in some cases, rounding will always produce some error, we make the arbitrary decision that being within one tenth of one percent is close enough, which means between 99.9% and 100.1%, inclusive. So with in this case, we should round to the nearest tenth of a percent.
Scales based on powers of 10: The most famous scale base on powers of ten in percentage, which really means "per 100". It is much more common to see "53% of the people agree with the president's plan" than ".53 of the people..." or "53 out of every 100 people...". Technically, all those phrases are saying the same thing, but percentage is the most popular.
One of the places where decimals are used for proportions is in the sports pages. A batting average in baseball (hits/at bats) is given as a percent to three decimal place, and likewise winning proportions (win/total games) are written as .xxx. If a batter has 27 hits in 92 at-bats, the batting average 27/92 = .293478261... is shortened to .293 and pronounced "two ninety three". Likewise, a team who has won 17 games and lost 5 will have a winning proportion of 17/22 = .77272727... = .773, and often stated as "team has a winning percentage of seven seventy three." Technically, this is a mistake, because "percentage" means out or 100. The correct word from the dictionary, which no one ever uses, is "permillage", which means out of 1,000. The team in question would have a winning percentage of 77/100, and a winning permillage of 773/1000.
In both of the cases from the sports pages, the greater number of place after the decimal is used to break ties. For example, a team with 14 wins and 4 losses is at .778, which is better than 17 wins and 5 losses, while 20 wins and 6 losses is at .769, so is slightly worse.
To get a number based on a power of 10 scale, you take the proportion and multiply by the power of ten, so it is either p*scale or p-hat*scale, depending on population or sample. Besides greater precision for breaking ties, sometimes we need greater precision because the proportions are so small.
When I ask a class what is the legal limit for blood alcohol while driving, invariably someone will say "point oh eight" and most people will agree. But .08 is wrong. .08 = 8%, and the correct answer is .08% = .0008. I don't blame the students. The number is badly represented and it is an easy mistake to make. Let's take a look at the number on other scales of 10.
.08 out of 100 is the same as
.8 out of 1,000 0r
8 out of 10,000 or
80 out of 100,000
80 parts out of 100,000 is a tiny proportion. To give an idea, ounce of pure alcohol mixed into ten gallons of blood would give you 78 parts out of 100,000, and most people have between a half gallon and a gallon and a half of blood in their body, between 4 and 12 pints. The amount of alcohol in a person's blood stream that is over the legal limit is about the same amount of alcohol as found in a capful of mouthwash used after brushing your teeth.
We will be dealing with much smaller proportions later in the class, where there are things that can be hazardous to your health at ranges measure in parts per billion, but for now, we will look at the per 100,000 scale for another type of statistic, measurements of mortality rates.
Here are the number of homicides in some local cities in 2007.
Oakland: 124 homicides
Richmond: 28 homicides
San Francisco: 98 homicides
Clearly, comparing these numbers is misleading, because we know these cities have very different numbers of citizens, so the standard way to measure these statistics is the per 100,000 population scale, which we find by the formula
f/n* scale
which in this case is
(# of homicides)/(city population) * 100,000
Oakland's population in 2007 is estimated at 415,000, Richmond at 106,000 and San Francisco at 825,000, so the murder rates on this standard scale are as follows
Oakland: 124/415000 * 100000 = 29.9
Richmond: 28/106000 * 100000 = 26.4
San Francisco: 98/825000 * 100000 = 11.9
So even though more people were murdered in San Francisco than in Richmond in 2007, the murder rate in Richmond was over twice as high, because Richmond has barely 1/8 of the population of San Francisco. (note: The trends for the three cities this decade are going in different directions. Oakland's murder rate is on the rise, while Richmond's is falling and San Francisco's has stayed about the same.)
Calculating proportions (probabilities): There are times when we will need to find new proportions from information previously calculated, either adding and subtracting old numbers or multiplying or dividing. It's best to use the fractional forms of the data when available, then round the answers after using the exact numbers instead of using answers that might have been rounded. Every time you use a rounded answer in a calculation, there is a change to increase the rounding error even more.
Labels:
data sets,
population,
reserved symbols,
rounding,
sample
Monday, June 22, 2009
Syllabus Summer 2009
Math 13: Introduction to Statistics Summer 2009 – Laney College
Instructor: Matthew Hubbard Text: none
Email: mhubbard@peralta.edu, profhubbard@gmail.com
website: budgetstats.blogspot.com
Office hours:
T-Th 9:25 to 9:55 am in G-201 (math lab)
M-W 3:15-3:45 pm in G-201 (math lab)
Wednesday 6-8 (math lab)
Scientific calculator required (TI-30X IIs or TI-83 recommended)
Important academic schedule dates
Last date to add, if class is not full: Sat., June 27Last date to drop class without a “W”: Thurs., July 2
Last date to drop class with a “W”: Wed., July 15
Holidays and professional development days that effect the Summer schedule:
NoneMidterm and Finals schedule:
Thurs., July 2 Midterm 1 (2 hours)Thurs., July 16 Midterm 2 (2 hours)
Thurs., July 30 (3 hours - comprehensive)
Grading Policy
Homework to be turned in: Assigned every Tuesday and Thursday, due the next class period(late homework accepted AT THE BEGINNING of class period after next, 2 points off)
Quizzes: Tuesdays and Thursdays weeks without midterms – no make-up quizzes
If arranged beforehand, make-up midterms can be given, but must be taken before the next class meeting.
The two lowest scores from homework and the lowest score from quizzes will be removed from consideration before grading.
Grading system
Quizzes * 25%Midterm 1 * 25%
Midterm 2 * 25%
Homework 20%
Final 30%
The lowest grade from Quizzes and the two Midterms will be dropped from the total.
Anyone getting a higher percentage score on the final than the weighted average of all grades combined will get the final percentage instead on the final grade, provided that student has not missed more than two homework assignments.
Academic honesty
Your homework, exams and quizzes must be your own work. Anyone caught cheating on these assignments will be punished, where the punishment can be as severe as failing the class or being put on college wide academic probation.Class rules
Cell phones and beepers turned off, no headphones or text messaging during classNo food or drink in class, except for sealable bottles. All empty bottles should be put in the recycling bins after class is over.
You will need your own calculator and handout sheets for tests and quizzes. Do not expect to be able to borrow these from someone else.
Student Learning Outcomes
1. Describe numerical and categorical data using statistical terminology and notation.
2. Analyze and explain relationships between variables in a sample or a population.
3. Make inferences about populations based on data obtained from samples.
4. Given a particular statistical or probabilistic context, determine whether or not a particular analytical methodology is appropriate and explain why.
Thursday, May 28, 2009
correlation practice
Here are three sets of seven numbers each. The x values are the number of points scored by Kobe Bryant in the playoff games against the Houston Rockets, the y values are the points scored by the Lakers in those same games and the z values are the difference between the Lakers' score and the Rockets' score in each game.
x: __32__40__33__35__26__32__33
y: __92_111_108__87_118__80__89
z: __-8_+13_+14_-12_+30_-15_+19
1. Find the correlation coefficients for all three pairs of number sets, rx,y, rx,z and ry,z.
2. What are the cut-off values for 95% confidence and 99% confidence for correlation?
3. Which of the pairs of sets has the highest absolute correlation and what confidence level does that correlation exceed?
Round all answers to three digits. Answers in the comments.
x: __32__40__33__35__26__32__33
y: __92_111_108__87_118__80__89
z: __-8_+13_+14_-12_+30_-15_+19
1. Find the correlation coefficients for all three pairs of number sets, rx,y, rx,z and ry,z.
2. What are the cut-off values for 95% confidence and 99% confidence for correlation?
3. Which of the pairs of sets has the highest absolute correlation and what confidence level does that correlation exceed?
Round all answers to three digits. Answers in the comments.
Wednesday, May 20, 2009
Topic for final exam
The final exam will be given at two times.
May 22: 8 a.m. to 10 a.m.
May 29: 10 a.m. to noon.
The final is comprehensive. You will need your yellow sheets, a calculator, scratch paper and a pencil.
The test will be four or five pages long. The amount from each part of the class will be
25%-30% from first exam
25%-30% from second exam
40-50% from after the second exam
On the list of topics below, any topic with an asterisk (*) means that though it might have been introduced before the first or second exam, it gets used throughout the class, so I don't necessarily count it as in the percentage of problem promised for each section.
Any topic in bold means you are expected to know how to get the answer without a formula or instructions being provided. In many cases, this means knowing how to use your calculator properly.
First exam
==========
frequency tables
stem and leaf plots
five number summary
box and whiskers
IQR and outliers for box and whiskers
Mean*, median*, mode, mid-range
parameter* and statistic*
population* and sample*
categorical data*
numerical data*
Bar charts
Pie charts
Line charts
Ogives
dotplots
percentage increase and decrease
contingency tables*
degrees of freedom*
conditional probability*
frequency and relative frequency
inclusion-exclusion
complementary event
order of operations*
Second exam through April 8
==========
standard deviation*
confidence intervals and margin of error
t-scores and z-scores*
raw scores, z-scores and percentages*
common critical values
Central Limit Theorem
Confidence of victory
After second exam
=======
Binomial coefficients and falling factorial
expected value of correct results
dependent and independent probabilities
Classic and modern parimutuel
expected value of a game
exactly r correct out of n trials
Bayesian probabilities
Hypothesis testing:
null hypothesis, alternative hypothesis, type I error, type II error
test statistic
threshold for xx% confidence (one-tailed high, one-tailed low, two-tailed)
one sample testing
two samples testing
Correlation (rx,y and the equation of the line yp = ax + b)
If you have any specific questions or want to make time to talk to me before the final, send me an e-mail and we can make an appointment.
May 22: 8 a.m. to 10 a.m.
May 29: 10 a.m. to noon.
The final is comprehensive. You will need your yellow sheets, a calculator, scratch paper and a pencil.
The test will be four or five pages long. The amount from each part of the class will be
25%-30% from first exam
25%-30% from second exam
40-50% from after the second exam
On the list of topics below, any topic with an asterisk (*) means that though it might have been introduced before the first or second exam, it gets used throughout the class, so I don't necessarily count it as in the percentage of problem promised for each section.
Any topic in bold means you are expected to know how to get the answer without a formula or instructions being provided. In many cases, this means knowing how to use your calculator properly.
First exam
==========
frequency tables
stem and leaf plots
five number summary
box and whiskers
IQR and outliers for box and whiskers
Mean*, median*, mode, mid-range
parameter* and statistic*
population* and sample*
categorical data*
numerical data*
Bar charts
Pie charts
Line charts
Ogives
dotplots
percentage increase and decrease
contingency tables*
degrees of freedom*
conditional probability*
frequency and relative frequency
inclusion-exclusion
complementary event
order of operations*
Second exam through April 8
==========
standard deviation*
confidence intervals and margin of error
t-scores and z-scores*
raw scores, z-scores and percentages*
common critical values
Central Limit Theorem
Confidence of victory
After second exam
=======
Binomial coefficients and falling factorial
expected value of correct results
dependent and independent probabilities
Classic and modern parimutuel
expected value of a game
exactly r correct out of n trials
Bayesian probabilities
Hypothesis testing:
null hypothesis, alternative hypothesis, type I error, type II error
test statistic
threshold for xx% confidence (one-tailed high, one-tailed low, two-tailed)
one sample testing
two samples testing
Correlation (rx,y and the equation of the line yp = ax + b)
If you have any specific questions or want to make time to talk to me before the final, send me an e-mail and we can make an appointment.
Monday, May 18, 2009
Class notes for 5/18: Regression and correlation
When we have a data set, sometimes we collect more than one variable of information about the units. For example, in our class survey, among the numerical variables were the height in inches, the GPA, the opinion about the difficulty of the class, age and average hours of sleep per night.
A question about two variables is if they are related to one another in some simple way. One simple way is correlation, which can be positive or negative. Here is a general definition of each.
Positive correlation between two numerical variables, call them x and y, means that the high values of x tend to be paired with the high values of y, the middle values of x tend to be paired with the middle values of y and the low values of x tend to be paired with the low values of y.
The variables x and y show negative correlation if that the high values of x tend to be paired with the low values of y, the middle values of x tend to be paired with the middle values of y and the low values of x tend to be paired with the high values of y.
If we pick two variables at random, we do not expect to see correlation. We can write this as a null hypothesis, where the test statistic is rx,y, the correlation coefficient. The sign of low correlation is rx,y = 0. The values of rx,y are always between -1, which means perfect negative correlation, and +1, which means perfect positive correlation.
The seventh page of the yellow sheets gives us threshold numbers for the 99% confidence level and 95% confidence level for correlation given the number of points n. For instance, when n = 5, the thresholds are .878 for 95% confidence and .959 for 99% confidence. This splits up the numbers from -1 to 1 into five regions.
-1 <= rx,y <= -.959: Very strong negative correlation
-.959 < rx,y <= -.878: Strong negative correlation
-.878 < rx,y < .878: The correlation is not particularly strong, regardless of positive or negative.
.878 <= rx,y < .959: strong positive correlation
.959 <= rx,y <= 1: very strong positive correlation
Just like with any hypothesis test, we should decide the confidence level before testing. This is a two-tailed test, because whether correlation is positive or negative, the relationships between number sets can often give us vital scientific information.
There is an important warning: Correlation is not causation. Just because two number sets have a relation, it doesn't mean that x causes y or y causes x. Sometimes there is a hidden third factor that is the cause of both of the things we are looking at. Sometimes, it's random chance and there is no causative agent at all.

Here is a set of five points, listed as (x,y) in each case.
(1,1)
(2,2)
(3,4)
(4,4)
(6,5)
As we can see, the points are ordered from low to high in both coordinates, so we expect some correlation. If we input the points into our calculator, we get a value for r (which is the same as rx,y) of .933338696..., which is strong positive correlation, but not very strong positive correlation. Assuming the 95% confidence level is good enough for us, we can use the a and b variables from out calculator to give us the equation of the line
yp = .797x + .649
This is called the predictor line (that's where the p comes from) or the line of regression or the line of least squares. Any such line for a given data set has two important criteria it meets. It passes through the centroid (x-bar, y-bar), the center point of all the data, and it minimizes the sum of the absolute values of the residuals, which is |y - yp| for all points.
Let's find the absolute values of the residuals for each of the five points, using the rounded values of a and b.
Point (1,1): |1 - .797*1 - .649| = 0.446
Point (2,2): |2 - .797*2 - .649| = 0.243
Point (3,4): |4 - .797*3 - .649| = 0.96
Point (4,4): |4 - .797*4 - .649| = 0.163
Point (6,5): |5 - .797*6 - .649| = 0.431
As we can see, the point (3,4) is farthest from the line, while the point (4,4) is the closest. The centroid (3.2, 3.2) is exactly on the line if you use the un-rounded values of a and b, and even using the rounded values, the centroid only misses the line by .0006.
In class, we used five points, but the last point was (5,6) instead of (6,5). This changes the numbers. rx,y goes up to .973328527..., which is above the 99% confidence threshold. The formula for the new predictor line is
yp = 1.2x - .2
Where we see the difference in these two different examples is in the residuals.
Point (1,1): |1 - 1.2*1 + .2| = 0
Point (2,2): |2 - 1.2*2 + .2| = 0.2
Point (3,4): |4 - 1.2*3 + .2| = 0.6
Point (4,4): |4 - 1.2*4 + .2| = 0.6
Point (5,6): |6 - 1.2*5 + .2| = 0.2
The closest point is now exactly on the line, which is a rarity, but even the farthest away point is only .6 units away, closer than the farthest away on the line with the lower correlation coefficient.
As we get more points in our data set, we lower our threshold that shows correlation strength. This way, a few points that are outliers do not completely ruin the chances of the data showing correlation, though sometimes strong outliers can mess up the data set and the correlation coefficient gets so close to zero that we cannot reject the null hypothesis that the two variables are not simply related.
A question about two variables is if they are related to one another in some simple way. One simple way is correlation, which can be positive or negative. Here is a general definition of each.
Positive correlation between two numerical variables, call them x and y, means that the high values of x tend to be paired with the high values of y, the middle values of x tend to be paired with the middle values of y and the low values of x tend to be paired with the low values of y.
The variables x and y show negative correlation if that the high values of x tend to be paired with the low values of y, the middle values of x tend to be paired with the middle values of y and the low values of x tend to be paired with the high values of y.
If we pick two variables at random, we do not expect to see correlation. We can write this as a null hypothesis, where the test statistic is rx,y, the correlation coefficient. The sign of low correlation is rx,y = 0. The values of rx,y are always between -1, which means perfect negative correlation, and +1, which means perfect positive correlation.
The seventh page of the yellow sheets gives us threshold numbers for the 99% confidence level and 95% confidence level for correlation given the number of points n. For instance, when n = 5, the thresholds are .878 for 95% confidence and .959 for 99% confidence. This splits up the numbers from -1 to 1 into five regions.
-1 <= rx,y <= -.959: Very strong negative correlation
-.959 < rx,y <= -.878: Strong negative correlation
-.878 < rx,y < .878: The correlation is not particularly strong, regardless of positive or negative.
.878 <= rx,y < .959: strong positive correlation
.959 <= rx,y <= 1: very strong positive correlation
Just like with any hypothesis test, we should decide the confidence level before testing. This is a two-tailed test, because whether correlation is positive or negative, the relationships between number sets can often give us vital scientific information.
There is an important warning: Correlation is not causation. Just because two number sets have a relation, it doesn't mean that x causes y or y causes x. Sometimes there is a hidden third factor that is the cause of both of the things we are looking at. Sometimes, it's random chance and there is no causative agent at all.

Here is a set of five points, listed as (x,y) in each case.
(1,1)
(2,2)
(3,4)
(4,4)
(6,5)
As we can see, the points are ordered from low to high in both coordinates, so we expect some correlation. If we input the points into our calculator, we get a value for r (which is the same as rx,y) of .933338696..., which is strong positive correlation, but not very strong positive correlation. Assuming the 95% confidence level is good enough for us, we can use the a and b variables from out calculator to give us the equation of the line
yp = .797x + .649
This is called the predictor line (that's where the p comes from) or the line of regression or the line of least squares. Any such line for a given data set has two important criteria it meets. It passes through the centroid (x-bar, y-bar), the center point of all the data, and it minimizes the sum of the absolute values of the residuals, which is |y - yp| for all points.
Let's find the absolute values of the residuals for each of the five points, using the rounded values of a and b.
Point (1,1): |1 - .797*1 - .649| = 0.446
Point (2,2): |2 - .797*2 - .649| = 0.243
Point (3,4): |4 - .797*3 - .649| = 0.96
Point (4,4): |4 - .797*4 - .649| = 0.163
Point (6,5): |5 - .797*6 - .649| = 0.431
As we can see, the point (3,4) is farthest from the line, while the point (4,4) is the closest. The centroid (3.2, 3.2) is exactly on the line if you use the un-rounded values of a and b, and even using the rounded values, the centroid only misses the line by .0006.
In class, we used five points, but the last point was (5,6) instead of (6,5). This changes the numbers. rx,y goes up to .973328527..., which is above the 99% confidence threshold. The formula for the new predictor line is
yp = 1.2x - .2
Where we see the difference in these two different examples is in the residuals.
Point (1,1): |1 - 1.2*1 + .2| = 0
Point (2,2): |2 - 1.2*2 + .2| = 0.2
Point (3,4): |4 - 1.2*3 + .2| = 0.6
Point (4,4): |4 - 1.2*4 + .2| = 0.6
Point (5,6): |6 - 1.2*5 + .2| = 0.2
The closest point is now exactly on the line, which is a rarity, but even the farthest away point is only .6 units away, closer than the farthest away on the line with the lower correlation coefficient.
As we get more points in our data set, we lower our threshold that shows correlation strength. This way, a few points that are outliers do not completely ruin the chances of the data showing correlation, though sometimes strong outliers can mess up the data set and the correlation coefficient gets so close to zero that we cannot reject the null hypothesis that the two variables are not simply related.
Labels:
class notes,
correlation,
predictor line,
regression
Subscribe to:
Posts (Atom)